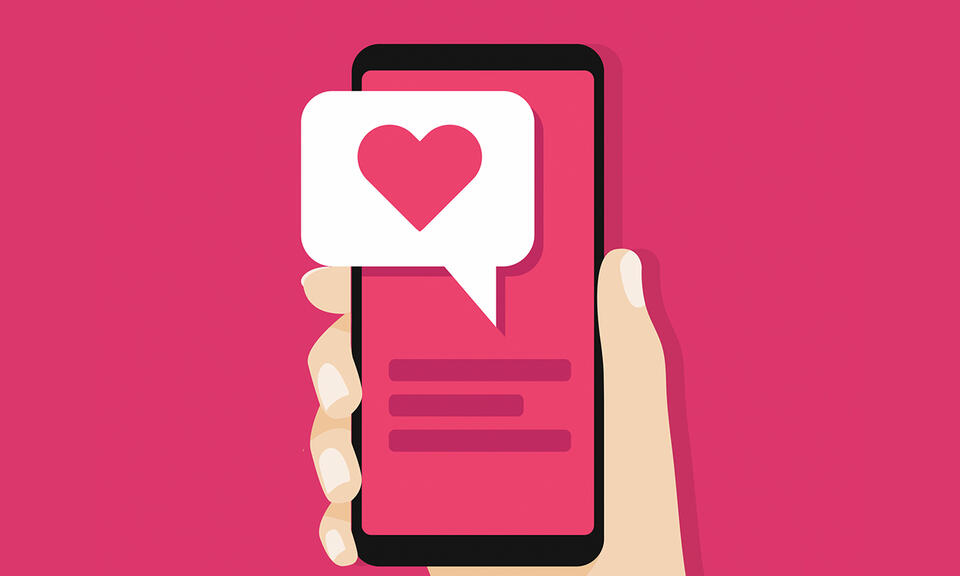
Written by
Published
Category
Key topics
Online dating can be frustrating and time-consuming, but not anymore. We’ve developed new algorithms that make finding your perfect match easier and more enjoyable. Here’s how we did it
Finding love is difficult enough without having to fathom how well dating services are doing their job of providing the perfect match. Today, this delicate task is one often left to algorithms – the “secret sauce” of hundreds of dating platforms serving millions of people around the world. But match rates across the online dating sector are often dismal. Luckily, for the many individuals who bemoan their unsuccessful sorties into the dating market, there is good news.
We’ve delved into the inner workings of algorithms used by a leading dating app and overhauled the technology – with happy results. By creating new algorithms more tailored to the individual, we’ve succeeded in increasing the number of matches by close to 30 per cent. This has wider implications for any businesses that rely upon algorithms to match customers in a two-sided market place.
How we cracked the code of dating app algorithms
Online dating is today worth $12 billion worldwide, and it’s one of the most common ways people meet their partners: in the US, some 65 per cent of same sex couples, and 39 per cent of heterosexual couples met online, research from 2018 shows. After lockdowns eased, dating app downloads soared.
But companies guard the finer details of the proprietary technology used to suggest potential dates. On the leading US platform with whom we collaborated, both users must declare they like each other for a match to be made. But most platforms don’t run every potential candidate past every other user – that would simply exhaust them. Instead, they limit the number of profiles a user sees; our partner shows a maximum of nine a day to each of its 800,000 users.
The more matches individuals have achieved in the recent past, the less likely they are to like profiles in the near future
By unpicking data held by our industry partner, we were able to understand the workings of their algorithm. This company uses profiles created by the individuals when they sign up – giving information about their age, gender, height, religion, education, location and more. They can also declare their preferences. When tastes converge, there’s a match. Note this model differs from the decentralised algorithms deployed by apps such as Tinder, which learn from users’ online behaviour.
The three keys to better dating matches
What we’ve done is to rejig the workings of this algorithm to bring in three major changes.
Firstly, we have succeeded in tailoring choices so that they are more personalised and granular. Currently, this platform applies a filter based on the user’s stated preferences and the platform’s broad assumptions – people with a degree will only be interested in others with a similar education, or of a similar height, or ethnicity, or interest in music for instance. But love may be hiding in unexpected places. Our algorithm allows for more nuance and different possibilities.
We’ve succeeded in increasing the number of matches by close to 30 per cent
We’ve also taken into account whether any "like" will be reciprocated. Half a match isn’t enough. Because our algorithm can also account for the recipient’s tastes, it makes a more judicious daily selection, making better use of the limited slots available for potential profiles, and increasing the chances of a match. Remember that individual users only see a limited number of profiles a day.
Our third innovation has been to understand and account for what we call "the history effect", which looks at a user’s recent activity on the app. The more matches that individuals have achieved in the recent past, the less likely they are to like profiles in the near future. What they experienced on the platform in their previous session will affect how they behave in the next, and we adjust for that. This may be down to feeling overwhelmed by the number of potential matches, or indeed there may be other factors at play. The more likes they get, for instance, the more attractive they might feel, and the more picky they may become.
How our algorithm performed in the dating market
Testing our algorithm in the real world is fraught with difficulties. These are real people seeking real relationships and we must tread carefully. We can’t dictate how they will react, and there’s no way to conduct a precise controlled experiment with like-for-like individuals.
But we were able to put the algorithm through its paces in a number of ways: firstly with a simulation using the platform’s data from activity of selected users based in Houston, Austin and Dallas – the three largest cities in Texas – over six months. It must be noted that all our experiments took place among heterosexual daters, who represent more than nine in 10 users in these environments. By simulating how individuals would react by using our algorithm, we were able to show a marked rise in the number of matches – enough to convince the dating platform to try it out in the real world.
Our algorithm allows for more nuance and different possibilities
And there, results were even more pronounced. We were able to test the new algorithm on individuals who used the app over a week in Houston. To gauge its performance, we compared the dating activity in very similar conditions in Dallas and Austin over the same time – and we saw a significant rise in the number of matches between Houston daters. In all, these experiments revealed a rise of at least 27 per cent.
Not only is this great news for those trying their luck in the dating market, it’s good news for companies seeking to improve their search and recommendation options. Our algorithmic experiments are broad enough to allow this innovation to be deployed in other two-sided markets, such as ride hailing apps and online work platforms. More personalised technology, better knowledge of the other party, and more oversight of recent online activity makes for success in dating, and elsewhere.
This article draws on findings from "Improving Match Rates in Dating Markets Through Assortment Optimization" by Ignacio Rios (The University of Texas at Dallas), Daniela Saban (Stanford University) and Fanyin Zheng (Imperial College London).